Análisis de regresión aplicado
Palabras clave:
Estadística aplicada, Análisis de regresiónSinopsis
El análisis de regresión es una herramienta de la inferencia estadística aplicada que permite ajustar conjunto de datos proveniente de trabajos de investigación con propósitos de predicción. En esta obra se trata una diversidad de técnicas útiles en esta tarea y se proporcionan las estrategias que permiten la selección de las variables, el tamaño de muestra y el mejor modelo; aquél que explique de mejor manera la relación entre las variables estudiadas. Esta técnica es aplicable en cualquier área de las ciencias: agropecuarias, biológica, económica, salud, genética, matemáticas, del comportamiento humano, etc., donde los objetivos incluyan predicción de resultados futuros de la variable respuesta (dependiente).
Descargas
Referencias
Acton, F. S. (1959). Analysis of straight –line data. Nueva York. Dover.
Aitkin, M. A. (1974). Simultaneous inference and the choice of variable subsets in multiple linear regression. Technometrics. 16: 221 -227.
Aktar, M. y Prescott (1986). Response surface designs robust to missing observations. Communications in Statistics: Simulation and Computation 15: 354 -363.
Allen, D. M. (1971). The prediction sum of squares as a criterion for selecting
predictor variables. Reporte Técnico No. 23. Departamento de Estadística, Universidad de Kentucky.
Allen, D. M. (1971). Mean square error of prediction as a criterion for selecting variables. Technometrics 13: 469 – 475.
Allen, D. M. (1974). The relationship between variables selection and data augmentation and a method for prediction. Technometrics 16: 125 -127.
Allen, T., L. Yu, y Bernshteyn (2000). Low-cost response surface methods applied to the design of plastic fasteners. Quality Engineering 12(4): 583 -591.
Anderson, M. J. y P. J. Whitcomb (2004). RSM Simplified: Optimizing processes using response surface methods for design of experiments. University Park. IL: Productivity Press.
Andrews, D. F. (1971). A note on the selection of data transformation. Biometrika 58: 249 -254.
Ankenman, B. E., H. Liu, A. F. Karr, y J. D. Picka (2002). A class of experimental designs for estimating a response surface and variance components. Technometrics 44(1): 45-54.
Armitage, P. (1971). Statistical Methods in Medical Research. Oxford: Blackwell Scientific Publications. U.K.
Atkinson, A. C. (1985). Plots, transformations and regression. Oxford: Clarendon Press.
Barnett, V. D. (1970). Fitting straight lines – the linear functional relationship with replicated observations. Applied Statistics 19: 135 – 144.
Bartlett, M. S. (1947). The use of transformations. Biometrics 3: 39 -52.
Bartlett, M. S. (1949). Fitting a straight line when both variables are subject to error. Biometrics 5: 164 – 180.
Beale, E. M. L. (1970). Note on procedures for variable selection in multiple regression. Technometrics 12: 909 – 914.
Beale, E. M. L., y P. C. Hutchinson (1974). Note on constrained optimum regression. Applied Statistics 23: 208 -210. Bendel, R. B., y A. A. Afifi. (1977). Comparison of stopping rules in forward “stepwise” regression. Journal of the American Statistical Association 72: 46 – 53.
Benignus, V. A., Muller, K. E., Barton, C. N. y Bittekofer, J. A. (1981). Toluene levels in blood and brain of rats ans after respiratory exposure. Toxicology an Application. Pharmacology 61: 326 -334.
Berk, K. N. (1978). Comparing subset regression procedures. Technometrics 20: 1- 6. Berenblut, I. I., y G. I. Webb (1973). A new test for autocorrelation errors in the linear regression model. Journal of the Statistical Society, Serie B 35: 33 – 50.
Berkson, J. (1950). Are there two regressions? Journal of the American Statistical Association 45: 164 – 180.
Bisgaard, S. (1997). Why three-level designs are not so useful for technological applications. Quality Engineering 9(3): 545-550.
Blalock, H. M., Jr., ed. (1971). Causal models in the Social Sciences. Chicago: Aldine
Publishing Company.
Bliss, C. I. (1936). The Size Factor in Action of Arsenic upon Silkworms Larvae. Journal Experimental Bilboar. 13: 95 -110.
Bowden, D. C. (1970). Simultaneous confidence bands for linear regression models. Journal of the American Statistical Association 65: 413 – 421.
Box, G. E. P. (1966). Use and abuse of regression. Technometrics 8: 625 -629.
Box, G. E. P. (1957). Evolutionary operation: A method dor increasing industrial productivity. Applied Statistics 6(2): 81 – 101.
Box, G. E. P. y P. W. Tidwell (1962). Transformations of the independent variables. Technometrics 4: 531- 550.
Box, G. E. P. (1999 – 2000). The invention of the composite design. Quality Engineering 12(1): 119 – 122.
Box, G. E. P. y D. R. Cox (1964). An analysis of transformations (with discussion).
Journal of the Royal Statistical Society, Series B 26: 211 – 246.
Box, G. E. P. y D. W. Behnken (1960). Some new three-level designs for ths study of quantitative variables. Technometrics 2: 455 – 475.
Box, G. E. P. y N. R. Draper (1969). Evolutionary Operation. New York: Wiley.
Box, G. E. P. y N. R. Draper (1975). Robust designs. Biometricka 62: 347 – 352.
Box, G. E. P. y N. R. Draper (1982). Measures of lack of fit for response surface designs and predictor variable transformations. Technometrics 24: 1 – 8.
Box, G. E. P. y N. R. Draper (1987). Empirical model building and response surfaces. New York: Wiley.
Box, G. E. P. y J. S. Hunter (1957). Multifactor experimental designs for exploring response surfaces. Annals of Mathematical Statistics 28: 195 -241.
Box, G. E. P. y K. B. Wilson (1951). On the experimental attainment of optimum conditions. Journal of the Royal Statistical Society, Series B 13: 1 – 45
Brown, P. J. (1977). Centering and scaling in ridge regression. Technometrics 19: 35 – 36.
Bursztyn, D. y D. M. Steinberg (2001). Rotation designs for experiments in high bias situations. Journal of Statistical Planning and Inference 97: 399 – 346.
Carter, W. H., V. M. Chinchilli, R. H Myers, y E. D. Campbell (1986). Confidence intervals and an improved ridge analysis of response surfaces. Technometrics 28: 339 -346.
Carroll, R. J., y D. Ruppert (1985). Transformations in regression: A robust analysis. Technometrics 27: 1 – 12.
Carroll, R. J., y D. Ruppert (1984). Power transformations when fitting theoretical
models to data. Journal American Statistics Association. 79: 321 – 328.
Chapman, R. E. y K. Masinda (2003). Response surface designed experiment for door closing effort. Quality Engineering 15(4): 581 - 585.
Chatterjee, S. y A. S. Hadi (1988). Sensitivity Analysis in linear regression. New York.
Cheng, S. W. y C. F. J. Wu (2001). Factor screening and response surface exploration. Statistica Sinica 11: 553 -580.
Conniffe, D. y J. Stone (1973). A critical view of ridge regression. Statistic 22: 181 -187.
Cox, D. R. (1960). Regression analysis when there is priori information about supplementary variables. Journal of the Royal Statistical Society Serie B 22: 172 -176.
Cox, D. R. (1970). The Analysis of Binary Data. Londres: Methuen and Co. Ltd.
Cox, D. R. y N. Reid (2000). The Theory of the Design of Experiments. Boca Raton, FL. CRC Press.
Croarkin, C. y P. Tobias, eds (2002). NIST/SEMATECH e-Handbook of Statistical Methods, joint effort of the national institute of standards and technology and international SEMATECH.
Davies, R. B. y B. Hutton (1975). The effects of errors in the independent variables in linear regression. Biometrika 62: 383 391.
Del Castillo, E. (1997). Stopping rules for steepest ascent in experimental optimization. Communications in statistics: simulations an computation 26(4): 1599 -1615.
Del Castillo, E. y S. Cahya (2001). A tool for computing confidence regions on the stationary point of a response surface. The American Statistician 55(4): 358– 365.
Doehlert, D. H. (1970). Uniform shell designs. Applied Statistics 19: 231 -239.
Draper, N. R. y R. C. Van Nostrand. (1979). Ridge regression and James Stein estimators; Review and comments. Technometrics 21: 451 – 466.
Draper, N. R. y H. Smith (1981). Applied regression analysis. Segunda Edición. Nueva York. John Wiley and Sons.
Draper, N. R. (1985). Small composite designs. Technometrics 27: 173 – 180.
Draper, N. R. y J. A. John (1998). Response surface designs where levels of some factors are difficult to change. Australian and New Zeland Journal of Statistics 40: 487 -495.
Draper, N. R. y D. K. J. Lin (1990). Small response-surface designs. Technometrics 32: 187 – 194.
Durbin, J. (1970). An alternative to the bounds test for testing for serial correlation in least squares regression. Econometrica 38: 422 -429.
Durbin, J. (1969). Test for serial correlation in regression analysis based on the periodogram of least squares residuals. Biometrika 56: 1 – 15.
Durbin, J. y G. S. Watson (1950). Testing for serial correlation in least squares regression, I. Biometrika 37: 409 -428.
Durbin, J. y G. S. Watson (1951). Testing for serial correlation in least squares regression, II. Biometrika 38: 1 - 19.
Durbin, J. y G. S. Watson (1971). Testing for serial correlation in least squares regression, III. Biometrika 58: 159 – 178.
Edmondson, R. N. (1991). Agricultural response surface experiments based on fourlevel factorial designs. Biometrics 47: 1435 – 1448.
Ezekiel, M. y K. A. Fox (1959). Methods of correlation and regression analysis. Nueva York. John Wiley and Sons.
Fang, K. T. y D. K. J. Lin (2003). Uniform experimental designs and their applications in industry. In Handbook of statistics, Vol. 22, Chsp. 4. Amsterdam: Elsevier Science B. V.
Fang, K. T. y R. Mukerjee (2000). A connection between uniformity and aberration in regular fractions of two –level factorials. Biometrika 87: 193 -1989.
Folks, J. L. (1967). Straight line confidence regions for linear models. Journal of the American Statistical Association 62: 1365 – 1374.
Foraythe, A. B., L. Engelman, R. Jennrich, y P. R. A. May (1973). A stopping rule for variable selection in multiple regression. Journal of the American Statistical Association 68: 75 –77.
Freund, R. J. (1979). Multicollinearity etc., Some New Examples. Procedings of the statistical Computing Section, American Statistics Association, pág. 111 –112.
Furnival, G. M. (1971). All possible regressions with less computation. Technometrics 13: 403 -408.
Galton, Sir Francis (1885). Regression towards mediocrity in heredity stature. Journal of Anthropological Institute 15: 246-263.
Geisser, S. (1975). The predictive sample reuse method with applications. Journal of the American Statistical Association 70: 320 – 328.
Ghadge, S. V. y H. Raheman (2006). Process optimization for biodiesel production
from mahua (Madhuca indica) oil using response surface methodology. Bioresource Technology 97(3): 379 – 384.
Ghosh, S. y W. S. Al-Sabah (1996). Efficient composite designs with small number of runs. Journal of statistical planning and inference 53(1): 117 – 132.
Giesbrecht, F. G. y M. L. Gumpertz (2004). Planning, construction and statistical analysis of comparative experiments. Hoboken. NJ: Wiley.
Gilmour, S. G. (2006). Response surface desings for experiments in bioprocessing. Biometrics 62: 323 – 331.
Gilmour, S. G. y N. R. Draper (2003). Confidence intervals around the ridge of optimal response on fitted second-order-response surface. Technometrics 45: 333-339.
Gilmour, S. G. y N. R. Draper (2004). Response. Technometrics 46: 358.
Gioivannitti – Jensen, A. y R. H. Myers (1989). Graphical assessment of the prediction capability of response surface designs. Technometrics 31: 159 –171.
Goldberger, A. S. (1961). Stepwise least-squares: residual analysis and specification. Journal of the American Statistical Association 56: 998 -1000.
Gorman, J. W., y R. J. Toman (1966). Selection of variables for fitting equations to data. Technometrics 8: 27 – 51.
Graybill, F. A. (1976). Theory and Application of the Linear Model. Boston, Massachusetts. Duxbury Press.
Green, L. W. (1970). Manual for scoring socioeconomic status for research on Health Behaviors. Public Healt Rept. 85: 815 -827.
Gunst, R. F. y R. L. Mason (1979). Some considerations in the evaluation of alternate prediction equations. Technometrics 21: 55 - 63.
Gunst, R. F. y R. L. Mason (1980). Regression analysis and its applications. A data oriented approach. Nueva York. Marcel Dekker.
Hahn, G. J. (1977). Fitting regressions models with no intercept term. Journal of Quality Technology 9: 56-60
Hahn, G. J. (1973). The coefficient of determination exposed. Chemical Technology 9(2): 56 - 61.
Hahn, G. J. (1972). Simultaneous prediction intervals for a regression model. Technometrics 14: 203 -214.
Halperin, M. (1961). Fitting of straight lines and predictions when both variables are subject to error. Journal of American Statistical Association 56: 657 – 669.
Hardin, R. H. y N. J. A. Sloane (1991). Computer-generated minimal (and larger) response surface designs: (I) The sphere.
Hartley, H. O. (1959). Smallest composite designs for response surfaces. Biometrics 15: 611 – 624.
Hedayat, A. y D. S. Robson (1970). Independence stepwise residuals for testing homoscedasticity. Journal of the American Statistical Association 65: 1573– 1581.
Hemmerle, W. J. (1975). An explicit solution for generalizated ridge regression. Technometrics 17: 309 - 314.
Henderson, H. V. y P. F. Velleman (1981). Building multiple regression models interactively. Biometrics 37: 391 - 411.
Hill, A. B. (1971). Principles of Medical Statistics, Novena Edición. Nueva York: Oxford University Press.
Hinkley, D. V. (1975). On power transformations to symmetry. Biometrika 62: 101 -111.
Hoagling, D. C., y R. Welch (1978). The hat matrix in regression and ANOVA. American statistician 32: 17 – 22.
Hoerl, R. W. (1985). Ridge analysis 25 years later. The American statistician 39: 186-192.
Hocking, R. R., y R. N. Leslie (1967). Selection of the best subset in regression analysis. Technometrics 9: 531 – 540.
Hocking, R. R. (1972). Criteria for selection of a subset regression: which one should be used? Technometrics 14: 967 – 970.
Hocking, R. R. (1974). Misspecification in regression. American Statistician 28: 39 -40.
Hocking, R. R. (1976). The analysis and selection of variables in linear regression. Biometrics 32: 1 – 51.
Huang, L. Z., Y. Lu, Y. Yuan, F: Lü, y X. Bie (2006). Optimization of a protective medium for enhancing the viability of freeze-dried Lactobacillus delbrueckii subsp. Bulgaricus based on response surface methodology. Journal of
Industrial Microbiology and Biotechnology 33(1): 55 – 61.
John, J. A. y N. R. Daper (1980). An alternative family of transformations. Applied Statistics 29: 190 -197.
Kennard, R. W. (1971). A note on the Cp statistic. Technometrics 13: 899 – 900.
Khuri, A. I. (2003). Current modeling and design issues in response surface methodology: GLMs and models with block effects. In Handbook of Statistics, Vol. 22, Chap.6. Amsterdam: Elsevier Science B.V.
Khuri, A. I., ed. (2005). Response surface methodology and related topics. Washington, D.C. World Scientific.
Khuri, A. I. y J. Cornell (1996). Response surface. Design and Analysis, segunda edición. Nueva York: Marcel Dekker.
Kim, B. H. y C. C. Akoh (2005). Modeling of lipase-catalyzed acidolysis of sesame oil and caprylic acid by response surface methodology: optimization of
reaction conditions by considering both acyl incorporation and migration. Journal of Agricultural and Food Chemistry 53(20): 8033 – 8037.
Kleijnen, J. P. C., D. den Hertog, y E. Angün (2004). Response surface methodology´s steepest ascent and step size revisited. European Journal of Operational Research 159: 121 -131.
Kleinbaum, D. G., Kupper, L. L., y Morgenstern, H. (1982). Epidemiologic Research. Belmont, Calif. Lifetime Learning Publications.
Krasker, W. S. (1980). Estimation in linear regression models with disparate data points. Econometrica 48: 1333 – 1346.
Kvalseth, T. O. (1985). Cautionary note about R2. American Statistician 39: 279 -285.
Lewis, T., y Taylor, L. R. (1967). Introduction to Experimental Ecology. Nueva York. A. P
Lindley, D. V. (1968). The choice of variables in multiple regression. Journal of the Royal Statistical Society Serie B 30: 31 -53.
Lowe, C. W. (1974). Evolutionary operation in action. Applied Statistics 23(2): 218-226.
McCabe, G. P. (1984). Principal variables. Technometrics 26: 137 -144.
Mc Daniel, W. R. y B. E. Ankenman (2000). A response surface test bed. Quality and Reliability Engineering International 16: 363 – 372.
Mallows, C. L. (1973). Some comments on Cp. Technometrics 15: 661 – 675.
Mantel, N. (1970). Why step-down procedures in variable selection. Technometrics 12: 621 – 625.
Marquardt, D. W. y R. D. Snee (1975). Ridge regression in practice. American Statistician 29: 3 -20.
Mays, D. P. y S. M. Easter (1997). Optimal response surface designs en the presence of dispersion effects. Journal of Quality Technology 29: 59 -70.
Mee, R. W. (2001). Noncentral composite designs. Technometrics 43(1): 34 -43.
Mee, R. W. (2004). Optimal three-level designs for response surface in spherical
experimental regions. Reporte Técnico 2004-3, Departamento de Estadísticas, Operaciones y Ciencias de la Administración, Universidad de Tennessee.
Mobert, M. K., K. E. Markides y D. Bylund (2005). Multi-parameter investigation of
tandem mass spectrometry in a linear ion trap using response surface modeling. Journal of Mass Spectrometry 40(3): 317-324.
Montgomery, D. C., y E.A. Peck (1982). Introduction to linear regression analysis. Nueva York. John Wiley and Sons.
Morrison, d. F. (1976). Multivariate Statistical Methods. Tercera edición. Nueva York.
Mosteller, F., y Tukey, J. W. (1967). Data analysis and regression. Reading, Mass: Addison-Wesley Publishing Company, Inc.
Myers R. H. (1971). Response surface Methodology. Boston: Allyn and Bacon.
Myers, R. H. (1999). Response surface methodology – current status and future directions. Journal of Quality Technology 31(1): 30 -44.
Myers, R. H. y D. C. Montgomery (1995). Response surface methodology: Process and Product Optimization using Designed Experiments. Nueva York: Wiley.
Myers, R. H. y D. C. Montgomery (2002). Response Surface Methodology: Process
and Product Optimization using Designed Experiments. Segunda edición. Nueva York: Wiley.
Myers, R. H., D. C. Montgomery, y G. G. Vining, C. M. Borror and S. M. Kowalski
(2004). Response surface methodology: A retrospective and literature survey. Journal of Quality Technology 36(1): 53 – 77.
Nagasawa, S., Osano, S., y Kondo, K (1964). An analytical method for evaluating the
susceptibility of Fish species to an Agricultural Chemical. Japón. Journal Applied Entomology Zool. 8: 118 -122.
Neter, J., Wasserman, W., y Kutner, M. H. (1983). Applied linear regression models. Homewood, Ill.; Richard D. Irwin.
Nicolai, R. P., R. Dkker, N. Piersma y G. J. Van Oortmarssen (2004). Automated response surface methodology for stochastic optimization models with known variance. In proceedings of the 2004 Winter Simulation Conference,
pp. 491 – 499. The society for computer simulation international, San Diego, Cal.
Notz, W. (1982). Minimal point second order designs. Journal of Statistical Planning and Inference 6: 47 -58.
Obenchain, R. L. (1977). Classical F-test and confidence regions for ridge regression. Technometrics 19: 429 – 439.
Obenchain, R. L. (1978). Good and optimal ridge estimators. Annals of Statistics 6: 1111 – 1121.
Olkin, I. (1967). Correlation Revised. In Improving experimental design and statistical analysis, ed. Julian C. Stanley. Chicago.
Olkin, I. y Siotani, M. (1964). Asymptotic distribution functions of a correlation
matrix. Stanford University Laboratory for Quantitative research in education, Report No. 6, Stanford, California.
Packer, P. E. (1951). An approach to Watershed protection criteria. J. Forestry 49: 638 -644.
Peterson, J. J. (1993). A general approach to ridge analysis with confidence intervals.Technometrics 35: 204 -214.
Peterson, J. J., S. Cahya y E. del Castillo (2002). A general approach to confidence
regions for optimal factor levels of response surfaces. Biometrics 58: 422 – 431.
Peterson, J. J., S. Cahya y E. del Castillo (2004). Letter to the editor. Technometrics 46(3): 355 -357.
Peterson, J. J. y A. M. Kuhn (2005). Ridge analysis with noise variables. Technometrics 47(3): 274 -283.
Picard, R. R. y Cook, R, D. (1984) Cross- validations of regression models. Journal American Statistics Association 79: 575 – 583.
Pope, P. T., y J. T. Webster (1972). The use of an F-statistic in stepwise regression procedures. Technometrics 14: 327 - 340.
Rao, P. (1971). Some notes on misspecification in multiple regression. The American Statistician 25: 37 -39.
Rencher, A. C., y F. C. Pun (1980). Inflation of R2 in best subset regression. Technometrics 22: 49 -53.
SAS Institute Inc. 1985. SAS User´s Guide Statistics, Version 5. Cary, North Carolina.
Schatzoff, M., R. Tsao y S. Fienberg (1968). The efficient calculation of all possible regressions. Technometrics 10: 769 -779.
Schreiner, H. R., Gerogine, R. C., y Lawrie, J. A. (1962). New Biological effects of the gases of the helium group. Science 136: 653 -654.
Searle, S. R. (1971). Linear models. Segunda Edición. Nueva York: John Wiley and Sons.
Seber, G. A. F. (1977). Linear regression analysis. Nueva York: John Wiley and Sons.
Shapiro, S. S. y Wilks, M. B. (1965). An Analysis of Variance Test for Normality (complete samples). Biometrika 52: 591 - 611.
Silvey, S. D. (1969). Multicollinearity and imprecise estimation. Journal of the Royal Statistical Society Series B 31: 539 – 552.
Smith, G. y Campbell, F (1980). A critique of some ridge regression methods. Journal American. Statist. Assoc. 75: 74 – 81.
Snee, R. (1977). Validation of regression models: Methods and Examples. Technometrics 19: 415 – 428.
Snee, R. D. (1985). Computer –aided design of experiments- some practical experiences. Journal of Quality Technology 17(4): 222 -236.
Sprent, P. (1971). Parallelism and concurrence in linear regression. Biometrics 27: 440-444.
Steinberg, D. M. y D. Bursztyn (2001). Discussion of Factor Screening and response
surface exploration by S. W. Cheng and C. F. J. Wu. Statistica Sinica 11: 596-599.
Stevens, J. P. (1984). Outliers and influential data points in regression ridge. Psychology Bull. 95: 334-344.
Stigler, S. M. (1981). Gauss and the invention of least squares. Annals of Statistics 99: 465-474.
Suich, R. y G.C. Derringer (1977). Is the regression equation adequate – one criterion. Technometrics 19: 213-216.
Sztendur, E. M. y N. T. Diamond (2002). Extensions to confidence region calculations for the path of steepest ascent. Journal of Quality Technology 34(3): 289-296.
Tang, M., J. Li, L. Y. Chan y D. K. J. Lin (2004). Application of uniform design in the formation of cement mixtures. Quality Engineering 16(3): 461-474.
Timm, N. H. (1975). Multivariate analysis with applications in education and psychology. Monterrey, California. Brooks.
Thompson, S. J. (1972). The doctor-patient relationship and outcomes of pregnancy. Ph. D. dissertation. Department of Epidemiology, University of North Carolina.
Tuck, M. G., S. M. Lewis y J.I.L. Cottrell (1993). Response surface methodology and Taguchi: A quality improvement study from the milling industry. Journal of the Royal Statistical Society, Series C 42: 671-676.
Tukey, J. W. (1977). Exploratory data analysis. Reading, Mass. Addison – Wesley.P. C.,I.
Vázquez, M. y A. M. Martin (1998). Optimization of Phaffia rhodozyma continuous
culture through response surface methodology. Biotechnology and Bioengineering 57(3): 314-320.
Steinberg, D. M. y D. Bursztyn (2001). Discussion of Factor Screening and response surface exploration by S. W. Cheng and C. F. J. Wu. Statistica Sinica 11: 596 -599.
Stevens, J. P. (1984). Outliers and influential data points in regression ridge. Psychology Bull. 95: 334-344.
Stigler, S. M. (1981). Gauss and the invention of least squares. Annals of Statistics 99: 465-474.
Suich, R. y G.C. Derringer (1977). Is the regression equation adequate – one criterion. Technometrics 19: 213-216.
Sztendur, E. M. y N. T. Diamond (2002). Extensions to confidence region calculations for the path of steepest ascent. Journal of Quality Technology 34(3): 289-296.
Tang, M., J. Li, L. Y. Chan y D. K. J. Lin (2004). Application of uniform design in the formation of cement mixtures. Quality Engineering 16(3): 461-474.
Timm, N. H. (1975). Multivariate analysis with applications in education and psychology. Monterrey, California. Brooks.
Thompson, S. J. (1972). The doctor-patient relationship and outcomes of pregnancy. Ph. D. dissertation. Department of Epidemiology, University of North Carolina.
Tuck, M. G., S. M. Lewis y J.I.L. Cottrell (1993). Response surface methodology and Taguchi: A quality improvement study from the milling industry. Journal of the Royal Statistical Society, Series C 42: 671-676.
Tukey, J. W. (1977). Exploratory data analysis. Reading, Mass. Addison – Wesley. P. C.,I.
Vázquez, M. y A. M. Martin (1998). Optimization of Phaffia rhodozyma continuous culture through response surface methodology. Biotechnology and Bioengineering 57(3): 314-320.
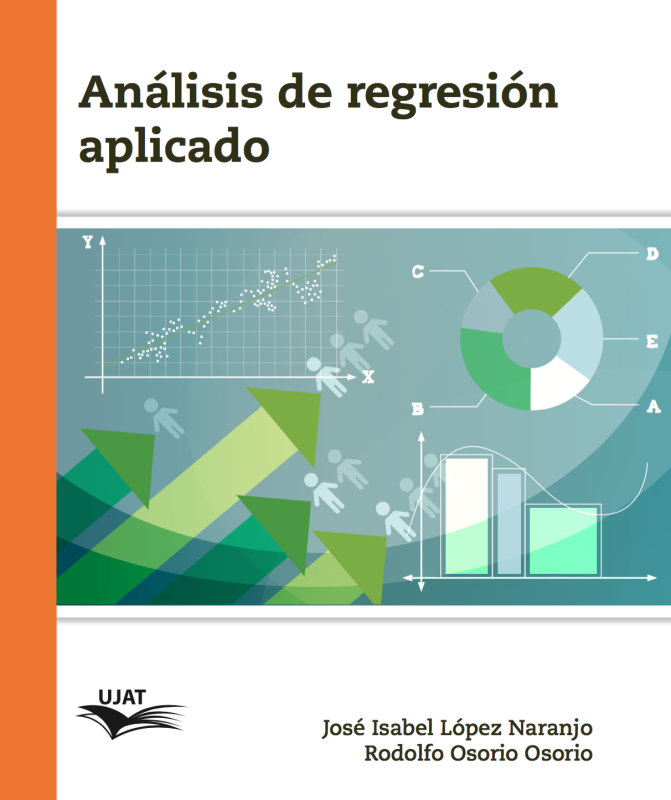